Doctoral thesis: use of smartphones sensor system to detect environmental anomalies
Publié le December 16 2019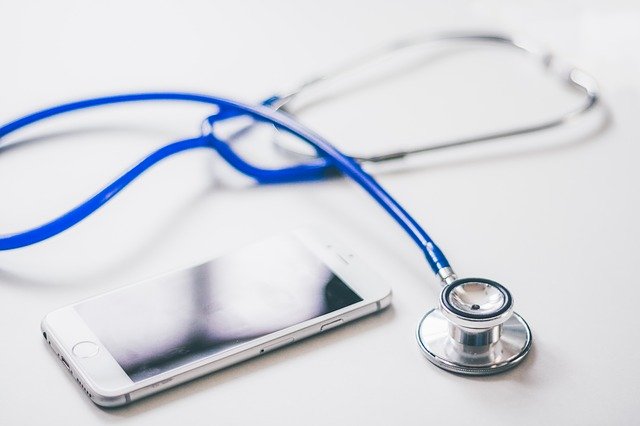
« Détection et agrégation d'anomalies dans les données issues des capteurs placés dans des smartphones »
TUESDAY, DECEMBER 17, 2019 at 2:30 p.m. - room A003 at Télécom SudParis 9 rue Charles Fourier - 91000 ÉvryJury members :
- M. Eric RENAULT, Professor, ESIEE, FRANCE - Supervisor
- Mme Selma BOUMERDASSI, Assistant professor, CNAM, FRANCE - Reviewer
- M. Hacène FOUCHAL, Professor, Université de Reims, FRANCE - Reviewer
- M. Viet Hai HA, Assistant professor, Université de Hué, Vietnam, VIET NAM - Co-supervisor
- Mme Thi Mai Trang NGUYEN, Assistant professor, Université Pierre et Marie Curie, FRANCE - Examiner
- Mme Véronique VÈQUE, Professor, CentraleSupélec, FRANCE - Examiner
Today, smartphones have become extremely popular and equipped with many sensors such as camera, microphone, GPS sensor, accelerometer, magnetometer, etc. Smartphone users connect more and more often to the Internet. As a result, there is a great deal of research into smartphone sensor systems in many areas of application. Our research aims at building a smartphone sensor system to detect environmental anomalies. We built the system model and improved the main components: anomaly detection method for the smartphone and method of synthesis of anomaly in the data center.
For anomaly detection methods, we rely on current research for the detection of traffic anomalies. In fact, there is a need for an efficient method that responds well to different environments and devices but consumes few resources. Therefore, we propose a method for anomaly detection based on the test of outliers in statistics. The improved algorithms have low complexity and use less memory. Our experiments on real data show that our methods adapt well to the different conditions in the case of the detection of road anomalies. The aggregation of anomaly data has not received much attention. Current research only stops at the simple grouping method based on distance. We developed a data integration method that makes it possible to find more precisely the location of anomalies.
We implemented two algorithms. A simple clustering algorithm that works cumulatively. This algorithm decomposes the data to increase speed and accuracy for anomaly location. Another algorithm makes it possible to find anomalies based on the search for probability density modes. We also propose a way to assign a weight to the found locations to assess reliability. Our simulations confirmed the effectiveness of this algorithm.